What is Social Media Sentiment Analysis? [Complete Guide]
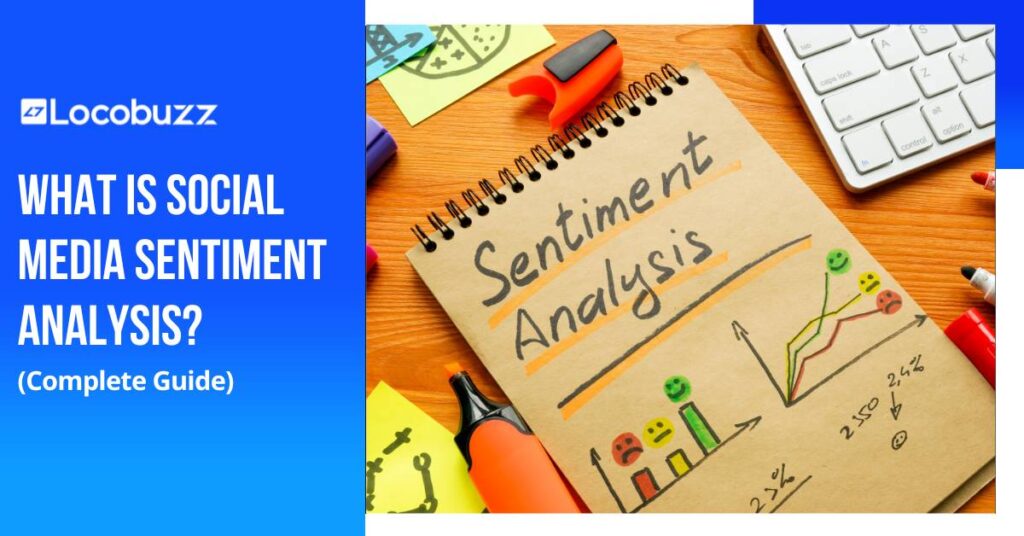
Understanding what people are saying online is crucial in today’s digital world and with over 4 billion people using social media globally, that’s a lot of opinions, feedback and discussions happening every minute.
But how do you make sense of this firehose of unstructured data? That’s where social media sentiment analysis comes in.
Sentiment analysis uses machine learning and natural language processing techniques to determine if what people are saying is positive, negative or neutral. It decodes this vast sea of emotions and viewpoints.
Did you know, over 70% of Fortune 500 companies now use some type of sentiment analysis tool?
This guide will break down the core components of social media sentiment analysis so you understand how it works and how you can leverage it for your brand.
What is Social Media Sentiment Analysis?
Social media sentiment analysis is the process of analyzing emotions, opinions and attitudes expressed on social platforms and through advanced text analytics, it decrypts massive amounts of consumer conversations happening every second across channels like Twitter, Facebook, Youtube and more.
The goal? To understand what people really think about your brand, products, competitors and current events. It surfaces valuable insights from unstructured social posts that other analytics can’t and by identifying positive, negative and neutral sentiments, businesses gain a deep perspective of their brand perception in real-time. This allows them to improve, respond faster and ultimately provide an experience that resonates with their target audience.
Types of Sentiment Analysis
Sentiment analysis can be categorized into different types based on the level of granularity and the approach used. Here are the main types of sentiment analysis:
- Polarity-Based Sentiment Analysis: This is the most basic form of sentiment analysis, which categorizes text into positive, negative, or neutral sentiments. It’s often used for quickly gauging the overall sentiment of a piece of text, such as a tweet or a product review.
- Aspect-Based Sentiment Analysis (ABSA): ABSA goes a step further by breaking down the text into different aspects or attributes and analyzing the sentiment for each aspect. For example, in a restaurant review, ABSA can separately evaluate sentiments about the food, service, ambiance, and price.
- Emotion Detection: This type of sentiment analysis identifies specific emotions expressed in the text such as happiness, sadness, anger, or surprise. It’s more nuanced than polarity-based analysis and can provide deeper insights into the emotional state of the writer.
- Intent Analysis: While not strictly sentiment analysis, intent analysis is closely related and often used in conjunction. It focuses on identifying the writer’s intention such as whether they are asking a question, expressing a desire, or making a complaint.
- Multilingual Sentiment Analysis: As businesses become more global, analyzing sentiment in multiple languages becomes crucial. Multilingual sentiment analysis involves developing models that can accurately assess sentiment across different languages and cultural contexts.
Each type of sentiment analysis serves different purposes and can provide unique insights depending on the application. By combining these approaches, businesses and researchers can gain a comprehensive understanding of the sentiments expressed in social media and other text data.
Decoding the Core Elements of Sentiment Analysis
Sentiment analysis is a cool way to understand how people feel about something by looking at what they write or say. It’s like a digital mood detector. Here’s a breakdown of its core elements:
- Understanding the Basics: Sentiment analysis is a tool that helps us figure out if a piece of text is positive, negative, or neutral. It’s like reading between the lines to catch the vibe of the words.
- Breaking Down the Text: The process starts by taking a piece of text and splitting it into smaller parts, like sentences or words. This helps the computer focus on each piece to understand the overall mood.
- Figuring Out the Feelings: The system then checks each part of the text against a list of words that have known emotions attached to them. Words like “happy” or “sad” give clues about the sentiment.
- Adding It All Up: After looking at all the pieces, the system adds up the positive and negative signals to decide the overall sentiment. It’s like weighing the good and bad to see which side is heavier.
- Dealing with Sarcasm and Context: One tricky part is understanding sarcasm or when the meaning changes based on the context. This is where advanced techniques come in to help the system get smarter.
- Putting It to Use: Sentiment analysis is super useful in many areas. Companies use it to see how people feel about their products, and it can even help track public opinion on social media.
So, sentiment analysis is a handy tool that helps us make sense of emotions in text, giving us a peek into the hearts and minds of people.
The Mechanism Behind Sentiment Analysis
Sentiment analysis is like a smart robot that reads text and figures out the emotions behind it. It’s a mix of language understanding and some cool math tricks. Here’s how it works:
- Natural Language Processing (NLP): This is the first step where the system breaks down the text into smaller pieces, like sentences and words. NLP helps the computer understand the structure of the language, like grammar and meaning.
- Machine Learning: This is where the magic happens. Machine learning uses algorithms to teach the computer how to recognize emotions in text. It’s like training a puppy to fetch; the more you practice, the better it gets.
- Algorithms and Models: There are different methods to do sentiment analysis. Some are simple, like looking up words in a list of positive or negative words. Others are more complex, like using neural networks, which are like a mini-brain inside the computer that can learn from lots of examples.
- Interpreting Emotions: Sentiment analysis tools use these algorithms to score the text. They might give a positive sentence a high score, a negative one a low score, and a neutral one somewhere in the middle. This helps us understand the overall mood of the text.
- Context and Sarcasm: One of the biggest challenges is figuring out the context and sarcasm. Sometimes, words can have different meanings depending on how they’re used. Advanced tools use more sophisticated techniques to catch these nuances.
- Applications: Sentiment analysis is super useful in many areas. Companies use it to see how customers feel about their products, politicians use it to track public opinion, and social media platforms use it to monitor trends.
In short, sentiment analysis is a blend of language understanding and machine learning that helps us decode emotions in text. It’s a powerful tool that’s becoming more and more important in our digital world.
Applications of Social Media Sentiment Analysis
Social media sentiment analysis is like a secret weapon for businesses. It helps them understand what people are saying about their brand, products, and services. Here’s how it’s used in different areas:
- Brand Reputation Monitoring: Companies use sentiment analysis to keep an eye on their reputation. By analyzing comments, tweets, and posts, they can get a sense of how people feel about their brand. It’s like having a pulse on public opinion, helping them stay ahead of any potential issues.
- Customer Feedback: Sentiment analysis is also great for digging into customer feedback. Businesses can see what customers love and what they’re not so happy about. This helps them make improvements, fix problems, and keep their customers happy.
- Market Research: When it comes to understanding the market, sentiment analysis is a game-changer. Companies can see what people are saying about their products compared to competitors. This gives them valuable insights into what’s working and what’s not, helping them make smarter decisions.
- Competitive Analysis: By keeping an eye on the competition, businesses can stay one step ahead. Sentiment analysis lets them see how their competitors are being perceived, allowing them to spot opportunities and threats.
- Crisis Management: In times of crisis, sentiment analysis can be a lifesaver. It helps businesses quickly understand how people are reacting to a situation. This allows them to respond appropriately, manage their reputation, and navigate through tough times.
In summary, social media sentiment analysis is a powerful tool for businesses. It helps them monitor their brand reputation, understand customer feedback, conduct market research, analyze competitors, and manage crises. With this tool, companies can make informed decisions and stay connected with their audience.
Benefits of Sentiment Analysis
understanding public sentiment has become a critical aspect of business strategy. Sentiment analysis, with its ability to sift through vast amounts of social media data, offers a range of benefits that can significantly enhance a company’s understanding of its audience, market positioning, and overall brand health. Let’s dive into some of the key advantages that sentiment analysis brings to the table.
- Improved Customer Insights: Sentiment analysis provides a deeper understanding of customer opinions and needs, helping businesses tailor their products and services to meet customer expectations.
- Enhanced Brand Reputation Management: By monitoring social media sentiment, companies can quickly identify and address negative feedback, protecting their brand image.
- Data-Driven Decision Making: Sentiment analysis offers actionable insights that can inform marketing strategies, product development, and other business decisions.
Competitive Advantage: Understanding the sentiment around competitors’ brands can help companies identify opportunities and threats in the market.
Increased Customer Engagement: By responding to customer sentiment in real time, businesses can foster stronger relationships with their audience and improve customer loyalty.
Challenges and Limitations of Sentiment Analysis
Sentiment analysis is a powerful tool, but it’s not perfect. One of the biggest challenges is understanding sarcasm. Sometimes, people say the opposite of what they mean as a joke or to make a point and for a computer, this can be tricky to figure out.
Context is another big challenge as the same word can have different meanings depending on how it’s used. For example, the word “sick” can mean ill, or it can mean awesome, depending on the context. This can make it hard for sentiment analysis tools to accurately interpret the sentiment.
Cultural nuances also play a big role. Words and expressions can have different connotations in different cultures and what’s considered positive in one culture might be neutral or even negative in another. This adds another layer of complexity to sentiment analysis.
Despite these challenges, sentiment analysis is still a valuable tool. However, it’s important to remember that it’s not always 100% accurate. Automated sentiment analysis can provide a general idea of the sentiment, but it might not catch all the nuances.
This is where human oversight comes in. By combining automated sentiment analysis with human review, businesses can get a more accurate picture of sentiment. Humans can pick up on sarcasm, context, and cultural nuances that the computer might miss. This helps ensure that the sentiment analysis is as accurate as possible.
Key Tools and Platforms
Sentiment analysis has become a vital tool in today’s digital world, and there are several popular platforms and tools available that cater to different needs. Here’s an overview:
- Natural Language Processing (NLP) Libraries: These are programming libraries designed to work with human language data. Examples include NLTK, SpaCy, and TextBlob. They offer various features for text processing, such as tokenization, part-of-speech tagging, and sentiment analysis.
- Machine Learning Frameworks: Tools like TensorFlow and PyTorch are used to build custom sentiment analysis models. They are more flexible and powerful, allowing for the development of sophisticated models that can handle complex language patterns.
- Cloud-based Platforms: Several cloud service providers offer sentiment analysis as part of their suite of machine learning services. These platforms provide APIs that can easily integrate sentiment analysis into applications. They are known for their scalability and ease of use.
- Social Media Monitoring Tools: There are tools specifically designed to monitor social media platforms for sentiment analysis. They can track mentions, hashtags, and keywords across various social networks and provide insights into public sentiment.
- Text Analytics Platforms: These platforms offer a range of text analysis capabilities, including sentiment analysis. They are often used in market research, customer feedback analysis, and other areas where large volumes of text data need to be analyzed.
Each of these tools and platforms has its own set of features and capabilities. When choosing a sentiment analysis tool, it’s important to consider factors such as the complexity of the text data, the level of accuracy required, and the specific use case.
Best Practices for Implementing Sentiment Analysis
Implementing sentiment analysis into social media strategies requires careful planning and execution. Here are some tips to ensure effectiveness:
- Define Clear Objectives: Before integrating sentiment analysis, it’s crucial to have clear goals. Understand what you want to achieve, whether it’s monitoring brand reputation, tracking customer satisfaction, or gaining insights into market trends.
- Choose the Right Tools: Select a sentiment analysis tool that suits your needs. Consider factors like accuracy, language support, and ease of integration with your existing social media platforms.
- Train and Customize: If possible, train your sentiment analysis model with data specific to your industry or brand. This can improve accuracy by adapting the tool to recognize industry-specific jargon and context.
- Monitor Continuously: Sentiment analysis should be an ongoing process. Regularly monitor and analyze social media data to stay updated on public sentiment and adjust your strategies accordingly.
- Respect Data Privacy: Ensure that your sentiment analysis practices comply with data privacy laws and ethical guidelines. Be transparent about how you collect and use data, and protect user privacy at all times.
By following these best practices, you can effectively integrate sentiment analysis into your social media strategies and gain valuable insights into your audience’s perceptions and emotions.
The Future of Sentiment Analysis in Social Media
The future of sentiment analysis in social media is exciting and full of potential. As technology advances, we can expect sentiment analysis tools to become more sophisticated and accurate. Artificial intelligence and machine learning will play a significant role in this evolution, enabling sentiment analysis to understand context, sarcasm, and subtle nuances in language better.
In the coming years, sentiment analysis will likely integrate more deeply with other technologies, such as chatbots and virtual assistants. This integration will allow for real-time sentiment analysis, enabling businesses to respond to customer emotions and feedback instantly.
We can also anticipate the rise of multilingual sentiment analysis, breaking down language barriers and providing a more global perspective on social media sentiment. Additionally, the use of sentiment analysis in detecting and managing mental health issues through social media interactions is a promising area of development.
Overall, the future of sentiment analysis in social media holds the promise of more accurate, real-time, and comprehensive insights into public sentiment, driving better decision-making and more personalized interactions in the digital world.
Conclusion
And there you have it! A complete guide to social media sentiment analysis, your digital compass in navigating the vast ocean of online opinions and emotions. As we’ve seen, sentiment analysis is not just about crunching numbers; it’s about understanding the heartbeat of your audience, capturing the pulse of public opinion, and responding with agility and empathy.
As we look to the future, the possibilities are as boundless as our imagination. With the continued evolution of AI and machine learning, sentiment analysis will become even more nuanced and insightful, offering a deeper dive into the human psyche and the ever-changing tides of social media sentiment.
So, whether you’re a brand guardian, a market researcher, or just someone fascinated by the interplay of technology and human emotion, sentiment analysis is your key to unlocking a treasure trove of insights. Embrace it, explore it, and let it guide your journey through the dynamic and ever-evolving landscape of social media.
Here’s to a future where we not only listen but truly understand the voices of the digital world!